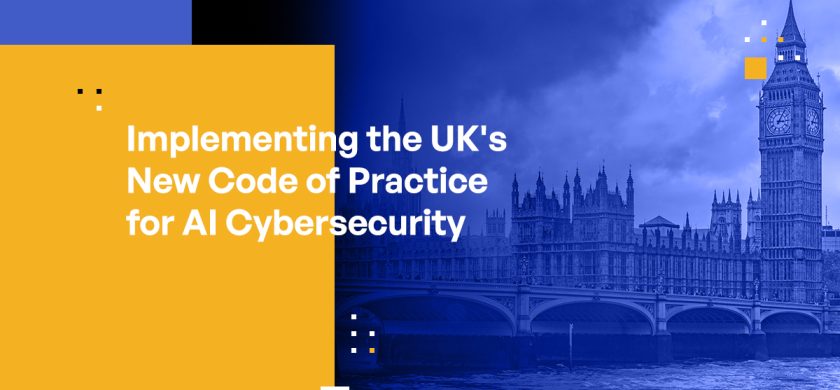
Understanding and Implementing the UK’s New Code of Practice for AI Cybersecurity: A Practical Guide
The UK government’s recently released Code of Practice for AI cybersecurity marks a significant milestone in addressing the unique security challenges posed by artificial intelligence systems. As organisations increasingly integrate AI into their operations, understanding and implementing this Code of Practice has become crucial for maintaining robust cybersecurity postures and ensuring compliance with emerging standards.
The UK’s Code of Practice for AI Cybersecurity, published in January 2025, represents a comprehensive framework designed to address the distinct security challenges presented by AI systems. With an overwhelming 80% endorsement rate from respondents to the Department for Science, Innovation and Technology’s Call for Views, this voluntary code establishes baseline security requirements that will inform future European Telecommunication Standards Institute (ETSI) standards.
For IT, risk, and compliance professionals, this framework provides essential guidance for securing AI systems throughout their lifecycle, from design to decommissioning. The Code’s comprehensive approach acknowledges the complex interplay between traditional cybersecurity measures and the unique challenges presented by AI technologies.
In this post, we’ll explore this new framework and provide actionable steps to help your organisation adhere to the framework’s guidelines to ensure your sensitive data is protected from AI ingestion.
Key Components of the Code of Practice
The Code of Practice comprises thirteen fundamental principles that span the entire AI system lifecycle. These principles form a comprehensive framework that addresses both traditional cybersecurity concerns and AI-specific challenges. Let’s examine these core components in detail.
Security Awareness and Design Principles
The Code begins by emphasising the importance of AI security awareness across organisations. It mandates regular security training programs specifically tailored to AI systems, requiring organisations to maintain current knowledge of emerging threats and vulnerabilities. This foundation of awareness supports the Code’s subsequent requirement for security-by-design approaches, where organisations must consider security alongside functionality and performance from the earliest stages of AI system development.
Threat Assessment and Risk Management
Central to the Code is the requirement for continuous threat evaluation and risk management. Organisations must implement comprehensive threat modeling that specifically addresses AI-related attacks, including data poisoning, model inversion, and membership inference. The Code emphasises that traditional risk management approaches must be adapted to account for AI-specific vulnerabilities and attack vectors.
Human Oversight and Responsibility
Recognising the unique challenges of AI system governance, the Code establishes clear requirements for human oversight. Organisations must implement technical measures that enable meaningful human supervision of AI systems, ensuring that outputs can be assessed and verified. This includes making model outputs interpretable and establishing clear lines of responsibility for system decisions.
Asset Management and Infrastructure Security
The Code mandates rigorous asset management practices, requiring organisations to maintain comprehensive inventories of AI-related assets, including models, training data, and associated infrastructure. It extends to infrastructure security, demanding specific controls for APIs, development environments, and training pipelines. This section particularly emphasises the need for secure access controls and environment separation.
Supply Chain Security and Documentation
Acknowledging the complexity of AI system development, the Code includes specific requirements for supply chain security. Organisations must implement secure software supply chain processes and maintain detailed documentation of model components, training data sources, and system changes. This includes requirements for cryptographic verification of model components and comprehensive audit trails.
Testing and Deployment Controls
The Code establishes robust requirements for testing and evaluation, mandating security assessments before system deployment. Organisations must conduct independent security testing and evaluate potential vulnerabilities in model outputs. These requirements extend to deployment practices, where organisations must provide clear guidance to end-users and maintain transparent communication about system capabilities and limitations.
Monitoring and Maintenance Requirements
Ongoing system monitoring forms a crucial component of the Code, requiring organisations to track system behavior, log activities, and analyse performance patterns. This includes monitoring for anomalies that might indicate security breaches or unexpected behavior changes. The Code also mandates regular security updates and patch management processes specific to AI systems.
End-of-Life Considerations
The final component addresses secure system decommissioning, requiring organisations to implement proper data and model disposal procedures. This includes specific requirements for transferring ownership of training data and models, ensuring that security considerations extend beyond the operational life of AI systems.
Through these components, the Code establishes a comprehensive framework that recognises the unique security challenges of AI systems while providing practical guidance for implementation. Each principle builds upon established cybersecurity practices while introducing specific requirements needed to address the distinct characteristics of AI technologies.
Key Takeaways
-
Comprehensive Framework for AI Security
The UK’s Code of Practice for AI Cybersecurity establishes a detailed framework addressing AI-specific security challenges across the entire system lifecycle. It provides essential guidance from secure design to decommissioning, emphasizing security-by-design and continuous risk management.
-
Unique AI Vulnerabilities and Operational Considerations
The Code targets vulnerabilities unique to AI systems, such as data poisoning, model obfuscation, and indirect prompt injection attacks. These challenges highlight the inadequacy of traditional security measures in the context of AI technologies, necessitating tailored approaches and roles for various stakeholders in the AI ecosystem.
-
Implementation Challenges
Organizations face complex implementation challenges, requiring significant resource allocation, stakeholder coordination, and ongoing monitoring. Establishing secure development environments, maintaining detailed documentation, and ensuring stakeholder alignment are critical for effective compliance.
-
Essential Actions for Compliance
Key actions for compliance include implementing comprehensive AI security training, developing risk management frameworks specific to AI, maintaining detailed documentation, and continuous system monitoring. These activities are crucial for adapting to emerging threats and ensuring system integrity.
-
Future Implications and Best Practices
Although the Code is currently voluntary, it is expected to influence future mandatory standards, representing best practices in AI security. Organizations are encouraged to start their compliance journey now to enhance security, operational efficiency, and reduce risk exposure, leveraging tools like the Kiteworks AI Data Gateway to support adherence to the Code’s requirements.
Understanding the Code of Practice and Its Objectives
The Code of Practice specifically targets AI systems, including those incorporating deep neural networks and generative AI. Unlike traditional software security frameworks, this code addresses unique AI-specific vulnerabilities and operational considerations across five key lifecycle phases: secure design, development, deployment, maintenance, and end of life.
What sets this framework apart is its recognition of AI’s distinct security challenges. Traditional software security measures, while necessary, prove insufficient for protecting against AI-specific threats such as data poisoning, model obfuscation, and indirect prompt injection attacks. The Code establishes a clear hierarchy of responsibilities, defining specific roles for various stakeholders within the AI ecosystem.
The framework recognises developers as organisations creating or adapting AI models and systems, while system operators take responsibility for deployment and ongoing management. Data custodians play a crucial role in controlling data permissions and maintaining integrity, working alongside end-users who actively engage with these systems. The Code also acknowledges affected entities – those individuals and systems indirectly impacted by AI decisions – ensuring a comprehensive approach to security and responsibility.
The Growing Need for AI Security Standards
The timing of the Code of Practice couldn’t be more crucial. As organisations rapidly adopt AI technologies, the attack surface and potential impact of security breaches have expanded dramatically. AI systems present unique security challenges that traditional cybersecurity frameworks don’t adequately address.
Data poisoning represents one of the most insidious threats to AI system integrity. Adversaries can manipulate training data in ways that compromise model integrity, potentially leading to biased or dangerous outputs. The challenge lies not just in preventing such attacks, but in detecting them, as the effects may only become apparent after deployment.
Model obfuscation presents another significant risk. Malicious actors may exploit model architectures to hide unauthorised functionalities or backdoors, creating security vulnerabilities that traditional testing protocols might miss. This risk becomes particularly acute as models grow more complex and their decision-making processes more opaque.
The rise of large language models and generative AI has introduced new vulnerabilities through indirect prompt injection attacks. These sophisticated attacks can manipulate AI systems into producing unauthorised or harmful outputs, bypassing traditional security controls and exploiting the very flexibility that makes these systems valuable.
Implementation Challenges
Organisations implementing the Code of Practice face several interconnected challenges that require careful consideration and strategic planning. The technical infrastructure requirements demand significant attention, as organisations must establish secure development environments while implementing comprehensive monitoring systems. These systems must maintain secure data pipelines while supporting robust testing frameworks throughout the AI lifecycle.
Stakeholder coordination presents another layer of complexity. Organisations must align responsibilities across different departments while ensuring clear communication channels remain open and effective. The management of third-party relationships becomes particularly crucial, as does the coordination of security responses across organisational boundaries.
Resource allocation requires careful balance. Organisations must invest in training and upskilling staff while also allocating resources to security tools and platforms. The maintenance of documentation systems and support for ongoing monitoring and updates demands sustained commitment and investment.
Essential Actions for Code of Practice Compliance
Successful implementation of the Code of Practice requires organisations to take several critical actions. First and foremost, organisations must establish comprehensive AI security training programs. The Code mandates regular security training that adapts to specific roles within the organisation. This training must evolve continuously as new threats emerge, ensuring staff maintain current knowledge of AI-specific security challenges and mitigation strategies.
Risk management forms another cornerstone of compliance. Organisations need to develop and maintain systematic threat modeling frameworks that specifically address AI-related vulnerabilities. This involves regular risk assessments that consider not only traditional cybersecurity threats but also AI-specific challenges such as model manipulation and data poisoning. The documentation of risk decisions and mitigation strategies becomes crucial for maintaining compliance and demonstrating due diligence.
Asset protection requires a sophisticated approach under the Code of Practice. Organisations must maintain comprehensive inventories of their AI assets, including models, training data, and associated infrastructure. Version control becomes particularly critical in AI systems, where changes to models or training data can have far-reaching implications for system security and performance. Access controls must be granular and context-aware, adapting to the specific requirements of AI system development and deployment.
Documentation emerges as a critical component of compliance. Organisations must maintain detailed records of their system architecture, security controls, and operational procedures. This documentation should include clear audit trails that track changes to models and systems, comprehensive incident logs, and detailed records of security assessments and remediation efforts.
Monitoring represents perhaps the most dynamic requirement of the Code. Organisations must implement continuous monitoring of their AI systems’ behavior, tracking performance metrics and watching for signs of compromise or manipulation. This monitoring should extend beyond traditional security metrics to include AI-specific indicators such as model drift and unexpected output patterns.
Implementation Roadmap
Organisations should approach Code of Practice compliance as a phased journey rather than a single project. The assessment phase typically requires one to two months, during which organisations thoroughly evaluate their current AI systems and security controls. This evaluation should identify gaps in current security measures and document existing processes, forming the foundation for subsequent planning.
The planning phase, typically lasting two to three months, focuses on developing a comprehensive implementation strategy. This includes resource allocation, training program development, and the establishment of monitoring systems. Organisations should pay particular attention to integrating new security controls with existing infrastructure during this phase.
Implementation represents the most intensive phase, usually requiring three to six months. During this time, organisations deploy security controls, conduct training programs, and establish documentation systems. The focus should remain on maintaining operational continuity while enhancing security measures.
Review and optimisation continue indefinitely, as organisations must regularly assess their security posture and update their controls in response to emerging threats. This ongoing process includes regular security assessments, policy updates, and continuous staff training.
Kiteworks Helps Organisations Adhere to the UK New Code of Practice With an AI Data Gateway
The UK’s Code of Practice for AI Cybersecurity represents a crucial step forward in securing AI systems. While implementation challenges exist, organisations that take a systematic approach to compliance will be better positioned to protect their AI assets and maintain regulatory compliance.
Success requires a comprehensive approach that combines technical controls, robust processes, and ongoing commitment to security. By leveraging tools like the Kiteworks AI Data Gateway, organisations can accelerate their compliance journey while ensuring the security and integrity of their AI systems.
Organisations should begin their compliance journey now, even though the Code is voluntary, as it will likely inform future mandatory standards and represents current best practices in AI security. The investment in compliance today will pay dividends in enhanced security, improved operational efficiency, and reduced risk exposure tomorrow.
The Kiteworks AI Data Gateway provides essential capabilities that align perfectly with the Code’s requirements. Through its secure AI data access functionality, the platform implements a zero-trust architecture that directly supports Principle 6 of the Code. This architecture ensures that every data access request is verified, regardless of its source, while maintaining the strict access controls required by Principle 5.
Governance and compliance capabilities built into the Kiteworks platform address several critical requirements of the Code. The system automatically enforces security policies while maintaining detailed audit logs that satisfy Principle 12’s documentation requirements. Real-time monitoring capabilities enable organisations to track and respond to potential security incidents promptly.
Data protection receives comprehensive treatment through end-to-end encryption and sophisticated access tracking. The platform maintains detailed records of all data access and transmission, enabling organisations to demonstrate compliance with data protection requirements while maintaining operational efficiency.
The platform’s support for Retrieval-Augmented Generation (RAG) proves particularly valuable for organisations implementing AI systems. By enabling secure data retrieval while maintaining strict access controls, Kiteworks allows organisations to enhance their model accuracy without compromising security. This capability becomes increasingly important as organisations seek to improve AI performance while maintaining compliance with the Code.
For more information about implementing the Code of Practice or to discuss how Kiteworks can support your compliance journey, contact our team of security experts.
With the Kiteworks Private Content Network organisations protect their sensitive content from AI risk with a zero trust approach to Generative AI. The Kiteworks AI Data Gateway offers a seamless solution for secure data access and effective data governance to minimise data breach risks and demonstrate regulatory compliance. Kiteworks provides content-defined zero trust controls, featuring least-privilege access defined at the content layer and next-gen DRM capabilities that block downloads from AI ingestion.
With an emphasis on secure data access and stringent governance, Kiteworks empowers you to leverage AI technologies while maintaining the integrity and confidentiality of your data assets.
To learn more about Kiteworks and protecting your sensitive data from AI ingestion, schedule a custom demo today.
Additional Resources
- Blog Post Kiteworks: Fortifying AI Advancements with Data Security
- Press Release Kiteworks Named Founding Member of NIST Artificial Intelligence Safety Institute Consortium
- Blog Post US Executive Order on Artificial Intelligence Demands Safe, Secure, and Trustworthy Development
- Blog Post A Comprehensive Approach to Enhancing Data Security and Privacy in AI Systems
- Blog Post Building Trust in Generative AI with a Zero Trust Approach