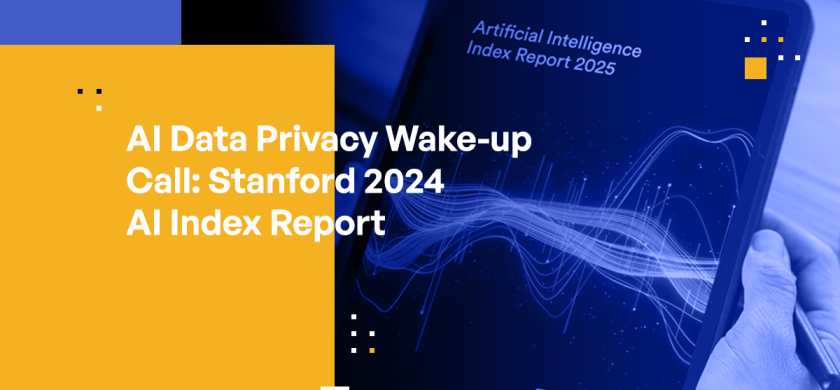
AI Data Privacy Wake-Up Call: Findings From Stanford’s 2025 AI Index Report
Organizations are facing an unprecedented surge in artificial intelligence-related privacy and security incidents. According to Stanford’s 2025 AI Index Report, AI incidents jumped by 56.4% in a single year, with 233 reported cases throughout 2024. These incidents span everything from data breaches to algorithmic failures that compromise sensitive information.
The findings reveal a disturbing gap between risk awareness and concrete action. While most organizations acknowledge the dangers AI poses to data security, fewer than two-thirds are actively implementing safeguards. This disconnect creates significant exposure at a time when regulatory scrutiny is intensifying across the globe.
For business leaders, the message is clear: the time for theoretical discussions about AI risk has passed. Organizations must now implement robust governance frameworks to protect private data or face mounting consequences—from regulatory penalties to irreparable damage to customer trust.
This analysis unpacks the most pressing findings from Stanford’s comprehensive report and offers practical guidance for strengthening your organization’s approach to AI data privacy and security.
Rising Tide of AI Risk: What the Numbers Tell Us
The AI Index Report paints a concerning picture of rapidly escalating risks. The 233 documented AI-related incidents in 2024 represent more than just a statistical increase—they signal a fundamental shift in the threat landscape facing organizations that deploy AI systems.
These incidents weren’t confined to a single category. They span across multiple domains:
- Privacy violations where AI systems inappropriately accessed or processed personal data
- Bias incidents resulting in discriminatory outcomes
- Misinformation campaigns amplified through AI channels
- Algorithmic failures leading to incorrect decisions with real-world consequences
Perhaps most concerning is the gap between awareness and action. While organizations recognize the risks—with 64% citing concerns about AI inaccuracy, 63% worried about compliance issues, and 60% identifying cybersecurity vulnerabilities—far fewer have implemented comprehensive safeguards.
This implementation gap creates a dangerous scenario where organizations continue deploying increasingly sophisticated AI systems without corresponding security controls. For business leaders, this represents a critical vulnerability that requires immediate attention.
The rising incident count isn’t merely academic—each event carries real-world costs:
- Regulatory fines that directly impact bottom lines
- Legal action from affected parties
- Operational disruptions during incident response
- Long-term reputation damage that erodes customer confidence
These statistics should serve as a wake-up call for organizations that have treated AI governance as a secondary consideration. The risks are no longer theoretical—they’re manifesting with increasing frequency and severity.
Public Trust in Decline: The Reputation Cost of Poor AI Governance
Customer trust is the foundation of any successful business relationship—and when it comes to AI and data privacy, that trust is eroding rapidly. The Stanford report reveals a troubling decline in public confidence, with trust in AI companies to protect personal data falling from 50% in 2023 to just 47% in 2024.
This erosion of trust doesn’t exist in isolation. It reflects growing public awareness about how AI systems use personal information and increasing skepticism about whether organizations are acting as responsible stewards of that data.
The trust deficit creates tangible business challenges:
- Customer reluctance to share information necessary for personalized services
- Increased scrutiny of privacy policies and data practices
- Growing preference for competitors with stronger privacy credentials
- Higher customer acquisition costs as skepticism rises
The report also highlights that 80.4% of U.S. local policymakers now support stricter data privacy rules—a clear indication that regulatory requirements will continue to tighten in response to public concern.
Forward-thinking organizations are recognizing that robust data privacy practices aren’t just about compliance—they’re becoming a competitive differentiator. Companies that demonstrate transparent, responsible data practices are increasingly able to convert privacy commitments into business advantages through enhanced customer trust.
The message is clear: organizations that fail to address the trust deficit face significant challenges in retaining and acquiring customers in an environment of growing privacy awareness.
Data Access Battlegrounds: The Shrinking Training Commons
A striking finding from the AI Index Report reveals a fundamental shift in how websites are responding to AI data collection. The percentage of websites blocking AI scraping has skyrocketed—increasing from just 5-7% to a remarkable 20-33% of common crawl content in a single year.
This dramatic rise reflects growing concerns about consent, copyright, and the appropriate use of publicly available information. Website owners are increasingly asserting control over how their content is used, particularly when it comes to training AI systems.
For organizations developing or deploying AI, this trend creates several immediate challenges:
- Shrinking access to high-quality training data
- Increased legal risk when using scraped content
- Questions about data provenance and consent
- Potential degradation in AI system performance
The implications extend beyond technical considerations. Organizations now face complex questions about data ethics and ownership:
- How can you ensure your training data was ethically and legally obtained?
- What consent mechanisms should be in place before using third-party content?
- How do you document data lineage to demonstrate compliance?
- What alternatives exist when traditional data sources become unavailable?
Organizations that fail to address these questions risk developing AI systems trained on potentially unauthorized data—creating significant legal and compliance exposure.
Moving forward, successful AI implementation will require thoughtful approaches to data sourcing. This includes obtaining explicit permission where required, developing fair compensation models for content creators, and exploring synthetic data alternatives that don’t rely on potentially restricted sources.
Regulatory Momentum: Preparing for the Compliance Storm
The AI regulatory landscape is expanding with unprecedented speed. According to Stanford’s findings, U.S. federal agencies issued 59 AI-related regulations in 2024—more than double the 25 issued in 2023. This regulatory surge isn’t limited to the United States; legislative mentions of AI increased by 21.3% across 75 countries globally.
This acceleration signals a new phase in AI governance, where theoretical frameworks are rapidly transforming into binding legal requirements. Organizations must now navigate a complex patchwork of regulations that varies by jurisdiction but shares common concerns about data privacy, security, and algorithmic accountability.
Particularly notable is the expansion of deepfake regulations, with 24 U.S. states now having passed laws specifically targeting synthetic media. These regulations focus on election integrity and identity protection—directly connecting to broader concerns about privacy and content authenticity.
For organizational leaders, this regulatory momentum requires proactive preparation:
- Regulatory mapping: Identify which AI regulations apply to your specific operations, customers, and geographic footprint
- Gap assessment: Compare your current practices against emerging requirements to identify areas needing improvement
- Documentation development: Create comprehensive records of AI development processes, data sources, and risk mitigation strategies
- Cross-functional governance: Establish teams that bring together legal, technical, and business perspectives
- Monitoring capabilities: Implement systems to track regulatory changes and assess their impact on your operations
The cost of regulatory non-compliance extends beyond potential fines. Organizations face business disruption if forced to modify or discontinue non-compliant AI systems, potential litigation from affected parties, and reputation damage from public enforcement actions.
Forward-looking organizations are approaching AI regulation not as an obstacle but as an opportunity to build more trustworthy, sustainable systems that align with emerging societal expectations and legal requirements.
Responsible AI: The Implementation Gap
Despite growing awareness of AI risks, the Stanford report reveals a troubling implementation gap in responsible AI practices. Standardized benchmarks for evaluating AI safety—such as HELM Safety and AIR-Bench—remain significantly underutilized, highlighting serious shortcomings in governance and validation procedures.
This gap is particularly concerning for privacy and security-critical deployments where algorithmic failures could compromise sensitive data or create security vulnerabilities.
While transparency scores for major foundation model developers have improved—rising from 37% in October 2023 to 58% in May 2024—this progress still falls well short of the comprehensive auditability required for regulatory compliance frameworks like GDPR or NIS2.
The implementation gap manifests in several key areas:
- Inadequate testing: Many organizations deploy AI systems without comprehensive evaluation against established safety benchmarks
- Limited documentation: Critical information about data sources, model limitations, and potential risks often remains undocumented
- Insufficient monitoring: Deployed systems frequently lack robust monitoring for performance degradation or unexpected behaviors
- Siloed responsibility: AI governance remains disconnected from broader security and privacy functions
Perhaps most concerning is the persistence of model bias despite explicit efforts to create unbiased systems. The report finds that even leading AI systems continue to exhibit biases that reinforce stereotypes—creating not only ethical concerns but also compliance risks under anti-discrimination laws.
Closing the implementation gap requires organizations to move beyond high-level principles to concrete action:
- Adopt structured evaluation frameworks that assess systems against established benchmarks
- Implement comprehensive documentation practices for all AI development and deployment
- Establish cross-functional review processes that include privacy, security, and compliance perspectives
- Develop continuous monitoring capabilities that track system performance in production environments
Organizations that successfully close the implementation gap gain significant advantages—from reduced compliance risk to greater stakeholder trust and more reliable AI performance.
AI-Driven Misinformation: A Threat to Data Integrity
The AI Index Report identifies a particularly troubling trend: the rapid growth of AI-generated misinformation. In 2024 alone, election-related AI misinformation was documented across a dozen countries and more than ten different platforms—representing an unprecedented scale of synthetic content designed to mislead.
This phenomenon extends far beyond politics. AI-generated misinformation now affects organizations across industries, creating new challenges for data integrity, brand protection, and information security.
The report details several concerning developments:
- Deepfakes that impersonate executives or manipulate financial information
- Altered campaign messages that undermine political processes
- Synthetic media that falsely represents products or services
- AI-generated content that circumvents traditional verification methods
For organizations, these developments create tangible risks:
- Damage to brand reputation from convincing forgeries
- Market volatility triggered by false information
- Customer confusion about authentic communications
- Erosion of trust in digital channels
Protecting against these threats requires a multi-faceted approach:
- Implement content authentication mechanisms that verify the source of communications
- Develop detection capabilities that can identify potentially synthetic content
- Establish rapid response protocols for addressing misinformation incidents
- Educate stakeholders about how to verify authentic communications
The growth of AI-driven misinformation represents a fundamental challenge to data integrity. Organizations must recognize that information security now extends beyond traditional data protection to include safeguarding the authenticity and accuracy of information itself.
Creating a Data-Secure AI Strategy: Action Plan
The findings from Stanford’s AI Index Report make clear that organizations need comprehensive strategies to address AI-related data privacy and security risks. This requires moving beyond reactive approaches to develop proactive frameworks that anticipate and mitigate potential harms.
Here’s a practical action plan for developing a data-secure AI strategy:
- Conduct a comprehensive AI risk assessment
- Inventory all AI systems and data sources currently in use
- Classify applications based on risk level and data sensitivity
- Identify specific threats to each system and its associated data
- Document regulatory requirements applicable to each application
- Implement data governance controls
- Apply data minimization principles to limit collection to necessary information
- Establish clear data retention policies with defined timelines
- Create granular access controls based on legitimate need
- Implement robust encryption for data in transit and at rest
- Adopt privacy-by-design approaches
- Integrate privacy considerations from the earliest development stages
- Document design decisions that impact data handling
- Conduct privacy impact assessments before deployment
- Build transparency mechanisms that explain data usage to users
- Develop continuous monitoring capabilities
- Implement systems to detect anomalous behavior or performance degradation
- Establish regular audit processes to verify compliance with policies
- Create feedback loops to incorporate lessons from monitoring
- Measure effectiveness of privacy and security controls
- Build cross-functional governance structures
- Form teams that include technical, legal, and business perspectives
- Define clear roles and responsibilities for AI oversight
- Establish escalation paths for identified issues
- Create documentation that demonstrates due diligence
Organizations that implement these steps position themselves not just for compliance but for competitive advantage in an environment of increasing scrutiny around AI data practices.
Future Outlook of AI Data Security and Compliance
The Stanford AI Index Report delivers a clear message: the risks of AI to data privacy, security, and compliance are no longer theoretical—they’re manifesting with increasing frequency and severity. Organizations face a critical choice between proactive governance and reactive crisis management. The statistics paint a compelling picture with a 56.4% increase in AI-related incidents in a single year, less than two-thirds of organizations actively mitigating known risks, public trust in AI companies declining from 50% to 47%, and regulatory activity more than doubling in the United States alone. These findings should serve as a wake-up call for organizational leaders. The time for abstract discussions about AI ethics has passed—concrete action is now required to protect sensitive data and maintain stakeholder trust.
Looking ahead, several trends are likely to shape the landscape: continued regulatory expansion with increasingly stringent requirements, growing public scrutiny of AI data practices and outcomes, further restrictions on data access as content creators assert control, and competitive differentiation based on responsible AI practices. Solutions like the Kiteworks Private Data Network with its AI Data Gateway offer organizations a structured approach to managing AI access to sensitive information, providing the security controls and governance needed to mitigate many of the risks highlighted in the Stanford report.
Organizations that recognize these trends and act decisively gain significant advantages—from reduced compliance risk to enhanced customer trust and more sustainable AI deployment. The path forward requires balancing innovation with responsibility. By implementing comprehensive governance frameworks, organizations can harness AI’s transformative potential while safeguarding the privacy and security of the data that makes it possible. The message from Stanford’s research is unambiguous: when it comes to AI data privacy and security, the time for action is now.
FAQs
The Stanford AI Index identifies several critical privacy risks, including unauthorized data access during training, the creation of synthetic identities from personal information, model inversion attacks that can extract training data, and the persistence of personal data in systems long after it should be deleted. The report specifically notes a 56.4% increase in privacy-related incidents in 2024.
Organizations should start by mapping applicable regulations to their specific AI applications, conducting gap assessments against these requirements, implementing standardized benchmarks like HELM Safety for systematic evaluation, documenting governance processes and data handling practices, and establishing continuous monitoring systems. The report indicates that transparency scores average only 58%, suggesting significant room for improvement.
Based on trends identified in the report, businesses should prepare for increased sectoral regulations targeting high-risk applications, expanded requirements for algorithmic impact assessments, stricter data provenance documentation, mandatory disclosure of AI use to consumers, and enhanced penalties for non-compliance. The 21.3% increase in legislative mentions across 75 countries signals accelerating regulatory momentum.
AI bias creates significant compliance exposure under anti-discrimination laws like the EEOC, GDPR Article 5, and similar regulations. The report documents persistent bias in leading AI systems despite explicit efforts to create unbiased models, highlighting the need for comprehensive testing, ongoing monitoring, documented mitigation efforts, and clear accountability structures to demonstrate due diligence.
Successful organizations implement structured processes that integrate responsibility without stifling innovation: establishing clear boundaries for acceptable use cases, creating stage-gate reviews that include ethical and compliance considerations, developing reusable components for privacy and security, building diverse development teams that identify potential issues early, and sharing best practices across the organization.
Organizations should begin with a comprehensive inventory of current AI applications and their associated data, conduct risk assessments prioritizing high-sensitivity systems, establish a cross-functional governance committee with clear authority, develop a framework adapting existing security and privacy controls to AI-specific challenges, and implement documentation practices that create accountability throughout the AI lifecycle.